How artificial intelligence can help farmers get more crops at lower costs
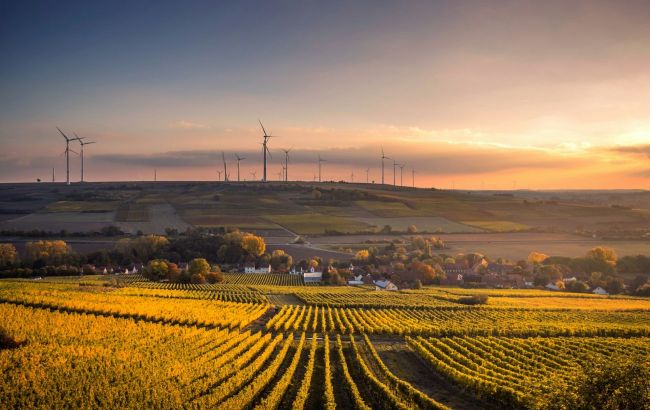
Artificial intelligence technologies are increasingly being implemented in agriculture, opening up new opportunities for farmers to increase efficiency. AI can not only reduce costs but also significantly increase yields.
RBC-Ukraine dwells on how artificial intelligence is changing the approach to farming and why it can be the key to sustainable and productive agriculture according to the World Economic Forum website.
Regenerative agriculture for future food security and sustainable food systems
Regenerative agriculture focuses on creating sustainable food systems by restoring soil and enhancing natural resources such as groundwater levels and biodiversity on farms.
Prioritizing soil restoration ensures long-term sustainability and increases yields through healthier, water-retaining soil. Regenerative agriculture, on the other hand, can reduce agricultural emissions by optimizing resource use.
At the farm level, this builds resilience, making farms better prepared to withstand environmental challenges and ultimately leading to more stable incomes.
Understanding role of digital technologies and artificial intelligence in agriculture
A little earlier than the global momentum towards regenerative agriculture, the digitalization of agriculture was emphasized. It offers benefits such as higher farmers' income, improved environmental outcomes, and better commercial viability when dealing with smallholder farmers.
Studies show that digital agriculture can increase the agricultural GDP of low- and middle-income countries by more than $450 billion, or 28 percent per year. The growing use of artificial intelligence (AI) in agriculture has further amplified these benefits for farmers.
For example, using AI and digital technologies, the World Economic Forum's AI for Agricultural Innovation initiative, in collaboration with the government of India, has supported chili pepper farmers to achieve a 21 percent increase in yields, a 9 percent reduction in pesticide use, and $800 in increased income from 40 acres of land per cycle.
Scenarios for the use of AI in agriculture (photo: The World Economic Forum)
Combining regenerative agriculture and artificial intelligence: promising applications
Many applications of artificial intelligence in agriculture have the potential to accelerate regenerative agriculture. Five such promising applications are highlighted.
Geospatial imagery for landscape planning
Scaling up regenerative agriculture often requires a landscape approach, focusing on the broader production sector rather than individual farms. This allows for holistic management/regeneration of natural resources.
AI models that use geospatial data can analyze changes in land cover and land use, soil health, and water availability over large tracts of land, helping to plan regenerative landscapes.
In Madhya Pradesh, the Center for Food Innovation, in partnership with the state government, is working with Skymet Weather to integrate geospatial imagery into landscape planning. The data collected will be further linked to financial instruments to better support farmers in adopting sustainable practices.
Digital expansion with the help of artificial intelligence
Regenerative agriculture relies on customized practices developed by research universities. Delivering these practices through extension agents is expensive, and the low ratio of agents to farmers leaves several landowners unserved.
Technological advances have improved the economics of disseminating such practices through digital channels. In addition, Large Language Models (LLMs) combined with Retrieval-Augmented Generation (RAG) models can make recommendations specific to farms based on localized data.
Additionally, AI-assisted language translations can cost-effectively provide delivery into local languages, making them more accessible in different regions.
Pest prediction to reduce pesticide use
The use of pesticides has been called a “global human rights issue” and regenerative agriculture programs are trying to gradually reduce their use.
Artificial intelligence solutions based on image recognition and hyperspectral imagery can provide both predictive and proactive pest detection, optimizing pesticide use.
Financial incentives through artificial intelligence
One of the obstacles to regenerative agriculture is the lack of financial incentives to adopt it. Financial incentives, such as payment for sequestered carbon (transformed carbon), are difficult due to the high costs of monitoring and payment.
However, recent pilots using sensors to measure soil health and smart contracts using artificial intelligence have made it possible to speed up payments and make them error-free and cost-effective.
Most carbon finance companies are using artificial intelligence models using geospatial data to remotely measure carbon sequestration.
The 100 Million Farmers Initiative uses similar innovative models to drive the transition. It provides a combination of financial and non-financial support for the transition to regenerative agriculture.
Thanks to artificial intelligence, it rewards both farmers and early investors. Blueprints for reproducing such financial models are available as part of the initiative.
Quick soil tests and program monitoring
AI-assisted soil testing provides a quick assessment of the health of the land, allowing for accurate decisions about the effectiveness of regenerative practices.
Additionally, AI geospatial models can be used to monitor practices such as crop rotation or cover cropping, which are typically difficult to monitor at scale.
Such analysis can also provide farmer segmentation, which will allow for individualized support to landowners at different levels of implementation.
Scaling artificial intelligence for regenerative agriculture
Today, several challenges need to be addressed to ensure that AI truly contributes to climate change action.
Reducing carbon footprint of AI
The growing demand for AI is leading to an increase in electricity usage, which results in emissions from tech companies. Reducing these emissions is crucial, and options that support this reduction, such as renewable energy and better data management, should be explored.
Optimize infrastructure and data structure
High-quality data is crucial for effective AI models, but agricultural data is often fragmented. Building a digital public infrastructure for data sharing can help reduce costs by allowing organizations to reuse and recycle data.
Harmonizing data collection through standards is necessary to ensure interoperability and increase the efficiency of data use. Another aspect is collecting data on farmers' practices and comparing it with other data sets on soil, water, etc. to create evidence that it works.
Structuring service delivery network at village level
It can be difficult for farmers to directly adopt AI-enabled technologies without any intermediary. Multi-stakeholder collaboration is needed to train and deploy village-level agents who can help assist farmers in delivering AI-enabled services.
As more agricultural data is accumulated and farmers become more familiar with the technology, the role of AI in regenerative agriculture will increase.
At the same time, with more data, the accuracy of existing solutions will improve. Therefore, to make the most of these advances, planning for the use of AI in the development of regenerative agriculture programs is crucial.